Anecdotes are great but what does the data say? Let us see some interesting insights that come out of analyzing 20M job postings over 16 month...<br>
Read More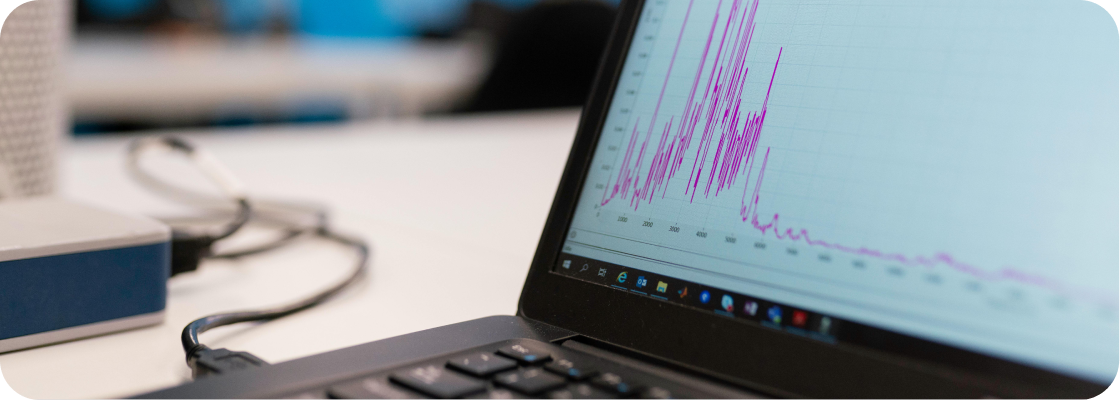
Three things to get right when scaling your data analytics operation

Amaresh Tripathy
Co-Founder and Managing PartnerI wrote earlier this year about how the heightened pressure and need for quick, accurate decision-making during the pandemic had helped to boost the profile of data analytics. All of a sudden, business leaders wanted more, and faster.
We’ve now reached an inflection point: the moment at which, as I pondered recently, we shift from the artistry and craft of the data discipline to functioning at scale.
Sure, innovation experiments are nice. But now it’s time to show whether they can work across the whole business, and prove that you can execute on a long-term vision.
Achieving this involves bringing talent, tech, and processes together. Below, I’ve broken down a few ways to get these three areas right.
Talent is about more than ticking all the boxes
There are few better examples of how to compile a team than special ops units. They follow a simple philosophy: hire the best, and train so that everybody knows their role, and all bases are covered.
It’s for a similar reason that most selective universities offer small class sizes: not just so that tutors can offer more attention to their students, but so that they can curate a classroom that has the best mix of approach and attitude so that every individual – and therefore the class as a whole – gets the most out of their studies.
These are principles worth borrowing from wherever possible when it comes to securing the right data and analytics talent.
Of course – because of time, budget, interest, location, or any other common constraint – you won’t always be able to cherry-pick so stringently. But following the right principles will reap rewards.
One less surmountable hurdle in this process, for now at least, is that data analytics is still a relatively new field. There aren’t that many people (yet) attending university to focus solely on this discipline.
This is changing, of course, but in the meantime, it’s worth identifying the qualities that will help you assemble the most coherent, cogent team – and hiring or pulling in existing staff accordingly.
A technology stack that can scale as fast as your ambitions demand
There are three key things to consider when it comes to assembling your stack.
First, accept that there is no established, standardized way of doing things. When you’re working to achieve scale, your circumstances will always be evolving. Accept that, and all the learning, mistakes, and serendipity it entails.
Second, look outside of your field to see who is scaling their technology successfully – and find out how they’re doing it.
Netflix added 26 million new paying subscribers in the first half of 2020. The previous year, it had added 28 million people for the whole year.
Managing this kind of growth would be nigh on impossible for Netflix to do itself; so it takes the smart option and has Amazon look after it, while the company focuses on what it does best – which includes, of course, making smart use of all that data.
While most of us think of Netflix as an entertainment destination, it is a highly data-driven business. The business uses data for everything from viewing recommendations and commissioning new content, all the way down to the cover art it shows you.
The point is, Netflix is both actively seeking scale and prepared for the extra load it places on the back end. A sudden influx of users isn’t going to hamper efforts in another area of the business.
This is the basis of the third important thing to consider with your tech stack: it won’t always be possible to have a neatly defined roadmap (or at least it won’t always be possible to stick to it), but so long as your tech decisions are always underpinned by the principle and direction of scale then you will make progress.
Scrap silos and adopt networked decision-making processes
It’s not possible to scale data analytics effectively when a business operates in silos.
Top-down, hierarchical structures that dole out orders and targets to teams working in their own top-down, hierarchical teams will always be limited in their ability to scale and provide new value successfully.
To make decision-making processes in your company scalable you need to view the organization as a network, with different disciplines and departments feeding into the decision-making process.
Again, Netflix provides a good example of how this can work.
The marketing staff making a big push for new subscribers during lockdown need to coordinate with the tech team who’ll be providing the necessary cloud support, and of course, the commissioners, producers and programmers will need to be looped in to provide a strong pipeline of new shows and films to keep those new subscribers coming back for more.
This is before you consider the complementary roles played by HR, finance, legal, or any number of other functions within the business.
It’s going to be our job, not just to ensure that teams across the organization have access to the right data at the right time, but that they have the skills to use that information effectively.
Because of this, the role of data analytics leaders will shift over the coming 12 months, focusing more and more on enabling networked decision-making.
In a very real sense, we have a key role to play in helping to optimize our organizations for decision making.
Just as HR and finance have built operating models based around business partners, so too will forward-looking data and analytics teams, working closer with colleagues to maximize the value our discipline can deliver.
Latest Post
The enterprise data stack is going through an inflection point and the battle lines are being redrawn. This time, to no surprise, the AI agenda in Enterprise going to determine the winners and losers...
Read More