Anecdotes are great but what does the data say? Let us see some interesting insights that come out of analyzing 20M job postings over 16 month...<br>
Read More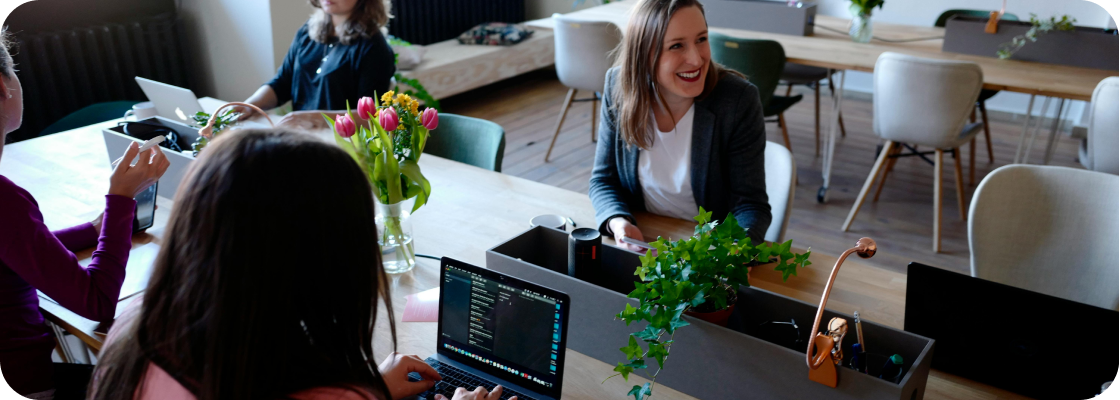
AI Enabled vs AI Native

Amaresh Tripathy
Co-Founder and Managing PartnerOur team at AuxoAI has been deep in building enterprise AI applications for last twelve months. They span across various functions (sales, service, support, pricing, R&D, etc.) across a few key industries.
Building probabilistic software systems that integrate data analytics, modeling, UX and deep engineering is a relatively novel area.
Here are our top learnings:
01. Many use cases don't need / cannot be solved by GenAI: Zero or very low tolerance of errors and no human in loop systems are not where you want to start your AI journey. Automation and ML models sometimes do a good enough job - no need to force fit the novel technology.
02. Most POCs aim too low: Designed to prove the technical capabilities vs. serving as a MVP that drives value. 90% of POCs are document Q&A vs. a bootstrap path that can drive critical metrics within the enterprise.
03. Game changing value from AI is in reimagining processes: Not an easy thing to do as it requires to think Future Back and then align it to Today Forward. Starting with the final 'reimagined process' vision and breaking it down into intermediate steps allows to deliver value with a regular cadence, while dealing with organizational change and resistance in bite sized chunks.
04. AI Applications are more than GenAI model outputs: Complex use cases are powered by an interaction between multiple agents exchanging information to process a user request. This interaction needs skills sets beyond simple prompt engineering to get the best output of the model. The ability to stitch all of it together in a reliable manner needs serious engineering.
05. Auditability is key: In RAG implementations, it is not just about getting an answer but the ability to transparently show the audit trail that highlights the steps and can connect to the content within the document(s).
06. Rich formatted documents: While basic RAG implementations are simple, documents with images, nested tables etc. make things complex very fast and need special handling
07. Insight across documents: Most high value use cases involve retrieving information from multiple documents where the final answer can come after multiple steps of processing of the different chunks of retrieved information and historical conversation.
Latest Post
The enterprise data stack is going through an inflection point and the battle lines are being redrawn. This time, to no surprise, the AI agenda in Enterprise going to determine the winners and losers...
Read More